The Full Circle of Data Governance - Data Mesh
- Philip Ho
- Feb 24
- 4 min read

For decades, companies have struggled with data.
First, businesses managed their own data—but it was fragmented and inconsistent.
Then, we centralised everything into warehouses and lakes, thinking it would fix the problem. But that just created bottlenecks.
Now, we’re decentralising again with Data Mesh.
But will this really solve data challenges, or are we just repeating history?
Researchers from Tilburg University, Eindhoven University, and the University of Salerno analysed 114 industry reports on Data Mesh. The results where mixed.
While Data Mesh helps scale data systems and remove bottlenecks, its success depends entirely on execution.
So, what do organisations need to do differently this time?
Figure 1 : The shift from decentralised data management (silos) → centralised warehouses/lakes → decentralisation via Data Mesh.
Lets first explore - What is Data Mesh?
Unlike traditional data warehouses, where one central team controls everything, Data Mesh decentralises data management—but in a structured way. It relies on 4 key principles:
Data Domains – Business areas like Marketing, Finance, and HR own and manage their own data.
Data Products – Instead of raw datasets, teams create ready-to-use, structured data products that solve real business problems.
Self-Serve Data Infrastructure – Teams can find, request, and use data without IT bottlenecks. Access should be effortless, and usage terms crystal clear.
Federated Governance – A decentralised yet standardised approach to security, compliance, and interoperability. Frameworks are distributed but enforced independently by each business.
In theory, this removes bottlenecks and makes data more accessible while keeping things structured. But theory and reality don’t always match up.
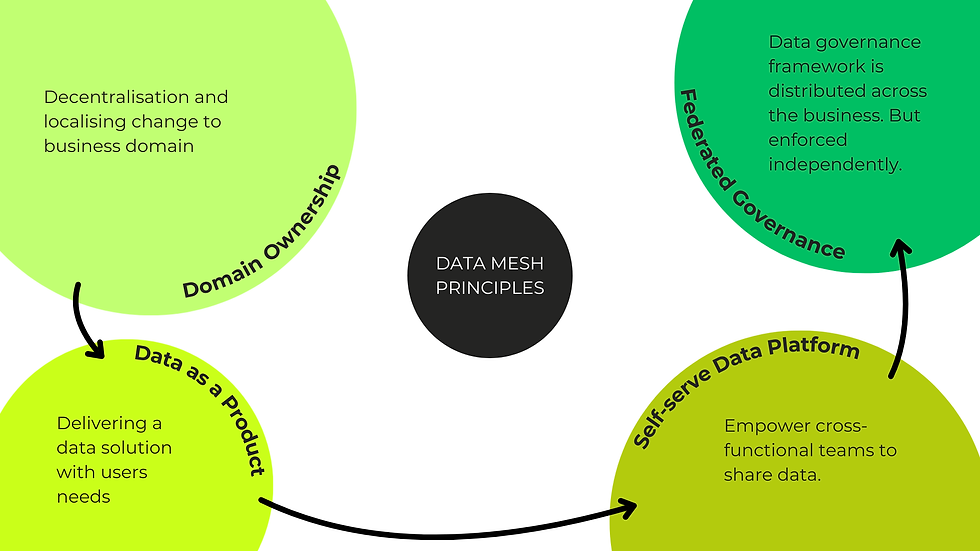
Figure 2: The concept of Data Mesh - domains, data products, and governance.
Will it work?
The research identified three major benefits:
Scalability – Eliminates bottlenecks from a single overloaded data team.
Faster data access – Business users can get the data they need without waiting for approvals.
Better data ownership – When teams are responsible for their own data, they take data quality more seriously.
But the research also warns of three major risks:
Data duplication – Without coordination, teams might recreate the same datasets, leading to waste and inconsistencies.
Lack of technical skills – Not every business unit has data engineering expertise to manage its own data effectively.
Inconsistency – If governance isn’t strong, decentralised data can become fragmented and unreliable.
Challenges in Implementing Data Mesh
Even with the right principles, Data Mesh isn’t an easy fix. Many organisations struggle with execution—and the biggest challenges come down to people, process, and speed.
Finding the Right Skills & Developing Them in the Business
Data Mesh shifts ownership from central IT teams to business domains—but many business teams aren’t ready to manage data. Business teams aren’t trained to manage data products—they’re used to relying on IT. IT needs to shift from “builders” to enablers—creating the right platforms, but not managing every data request. Data teams need to work more closely with business teams—understanding use cases, not just writing queries.
Defining the Actual Data Product
The biggest challenge in Data Mesh is clarity—what exactly is a data product? Many teams struggle to define what problem it solves, who owns it, and how it should be structured. If no one knows what the product is for, it won’t be useful. If ownership isn’t clear, maintenance will suffer. If schemas, definitions, and metrics aren’t standardised, every team will do things their own way.
Setting the Rules & Getting Agreement Across the Organisation
One of the biggest risks with Data Mesh is every team doing things their own way—creating chaos instead of efficiency. Teams want autonomy, but interoperability is crucial. Different teams may have conflicting priorities—Finance needs accuracy, Marketing needs speed. Governance rules must be flexible enough to work across all domains.
Balancing Governance: How Much Is Too Much? What Is Seamless?
The biggest mistake companies make is either too much governance or too little. Too much control—business teams lose autonomy, and bottlenecks return. Too little control—data becomes fragmented, redundant, and unreliable. The right balance is different for every company—some industries (e.g. finance) need strict oversight, while others (e.g. startups) can move faster.
The Speed of Execution: The Business Still Needs to Run
At the end of the day, Data Mesh isn’t just a technical shift—it’s an operational one. The biggest risk? Companies getting stuck in endless planning mode. Businesses still need to deliver results while transforming data management. IT teams may be resistant to losing control, slowing down implementation. If execution is too slow, teams will abandon Data Mesh and go back to old habits.
How to Execute Data Mesh Successfully
Organisations need to tackle these challenges while ensuring the business continues to operate smoothly. Upskilling business teams should be a priority—introducing hybrid roles like "Data Product Owners" who understand both business and technical needs. IT should transition into an enabler role, focusing on building platforms that make it easy for business teams to manage data independently. Clear definitions of data products, ownership, and governance policies must be established from the start, ensuring standardisation and interoperability across teams. Governance should act as a set of guardrails, not rigid roadblocks—policy-as-code can automate compliance without slowing teams down. Most importantly, organisations should start small—piloting the framework in high-impact domains, proving its value early, and gradually scaling across the business. If companies fail to execute efficiently, Data Mesh risks becoming another overhyped concept that never delivers real value.
References
Dehghani, Z. (2019). Data Mesh Principles and Logical Architecture. ThoughtWorks. Retrieved from martinfowler.com
Tilburg University, Eindhoven University, University of Salerno (2023). Data Mesh: A Systematic Gray Literature Review. Retrieved from [Academic Research Source]
Eckerson Group (2022). The Continuing Evolution of Data Management. Retrieved from [eckerson.com]
Data Mesh Learning Community. (2022). Best Practices in Implementing Data Mesh. Retrieved from [datamesh.community]
Comments