How AI Learns from Fake Data!
- Philip Ho
- Mar 9
- 3 min read
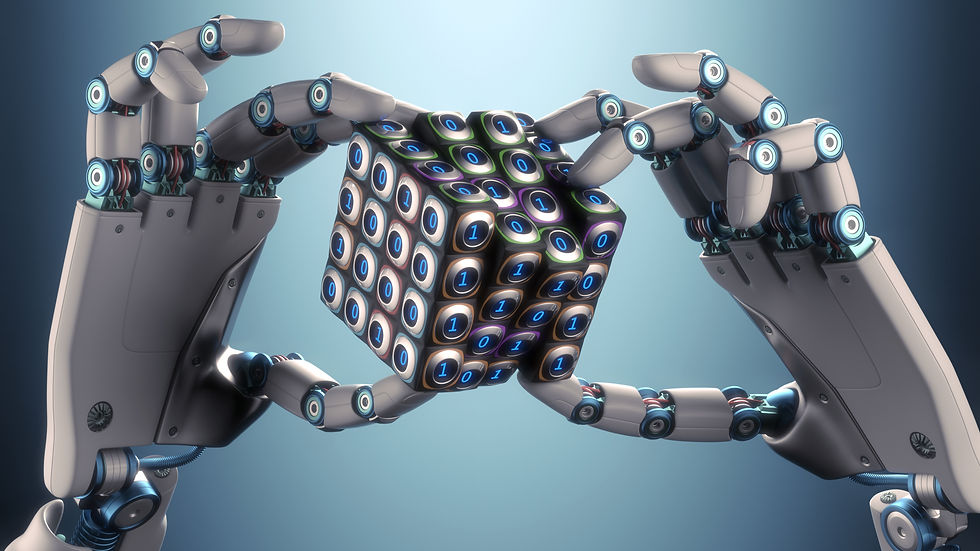
AI is only as good as the data it learns from. But what if there’s not enough data—or worse, what if it’s biased? That’s where synthetic data comes in. Synthetic data is here to level the playing field, making sure AI models can learn from diverse, unbiased, and privacy-safe datasets.
A study from the United Nations University explores how synthetic data could solve major AI challenges—like protecting privacy, filling data gaps, and reducing bias in AI models. This is especially important in regions where real-world data is limited or incomplete, such as in the Global South, where AI often lacks sufficient training data.
But synthetic data isn’t perfect. While it offers solutions, it also introduces risks—security vulnerabilities, potential bias amplification, and quality concerns. This week’s paper dives into these opportunities and challenges, along with recommendations on how to ensure synthetic data is used responsibly.
What is Synthetic Data?
Synthetic data is artificially generated data that mimics real-world data patterns without copying actual personal information. It’s used when real data is scarce, sensitive, or too costly to collect.
There are three main ways synthetic data is created:
Rule-Based Simulations – Using predefined conditions to generate structured data
Statistical Models – Learning from real data distributions and producing new data that follows similar statistical properties.
AI-Generated Synthetic Data – Techniques like Generative Adversarial Networks (GANs) and Large Language Models (LLMs) can create highly realistic synthetic images, text, and structured data.
Synthetic data is already used in healthcare, finance, and autonomous vehicle training, providing AI models with large-scale, privacy-safe training data. But how effective is it?
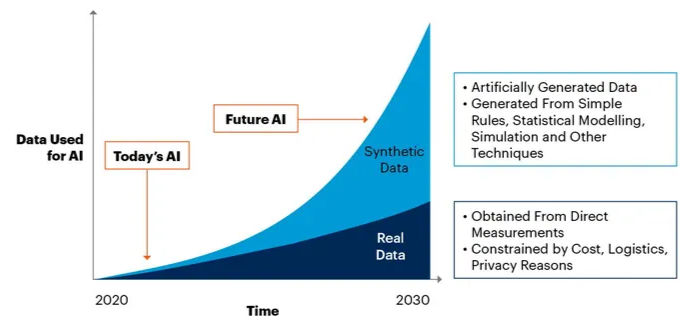
Key Benefits of Synthetic Data
Fixing Bias in AI Models
One of AI’s biggest challenges is bias—when models are trained on datasets that don’t reflect all groups. For example:
Facial recognition AI has had high error rates for women and people of colour due to biased datasets.
Loan approval systems may favour applicants from certain backgrounds if historical data is skewed.
Synthetic data helps fill these gaps, ensuring AI models learn from a broader, more balanced dataset rather than reinforcing existing inequalities.
Protecting Privacy & Meeting Regulations
AI models trained on real personal data raise concerns around privacy laws like GDPR. Synthetic data replaces real personal records with artificial ones, allowing companies to train AI without exposing sensitive details.
This is especially useful in:
Healthcare – Where patient records need strict privacy protection.
Financial Services – To train fraud detection models without leaking customer transactions.
Addressing Data Scarcity
The Global South often lacks large, high-quality datasets due to limited infrastructure, funding, or digitisation. Synthetic data can generate realistic economic, medical, and demographic datasets, allowing AI to be trained on more regionally relevant data.
The Risks & Challenges of Synthetic Data
Data Quality Issues
Not all synthetic data is equal. Poorly generated synthetic data can:
Misrepresent reality, leading to AI making poor decisions.
Lack real-world complexity, causing models to behave unpredictably in real-world applications.
Security Risks & Re-Identification
Even though synthetic data doesn’t contain real people’s details, attackers could potentially reverse-engineer patterns and infer private information.
If synthetic data closely resembles real-world patterns, it might be possible to identify individuals based on unique attributes.
AI models trained on synthetic data could memorize sensitive details, defeating the purpose of privacy protection.
Bias Amplification Instead of Reduction
If the real-world dataset used to create synthetic data is already biased, synthetic data can repeat and amplify those biases rather than fixing them.
For example: If a healthcare dataset underrepresents certain populations, synthetic data generated from it might continue to exclude key groups, leading to inaccurate medical predictions.
Making the Most of Synthetic Data
When done right, synthetic data can reduce bias, protect privacy, and fill data gaps—but poor implementation can lead to security risks and flawed AI models.
Here’s how to make the most of synthetic data:
Use diverse real-world data sources – Ensure a broad, representative dataset to prevent bias.
Leverage different AI models – No one-size-fits-all approach. Choose models based on your use case:
Generative Adversarial Networks (GANs) – Best for creating realistic images and text, used in medical imaging and deepfake detection.
Variational Autoencoders (VAEs) – Ideal for structured data generation, often used in fraud detection.
Large Language Models (LLMs) like GPT – Useful for generating synthetic text-based datasets in customer service AI.
Agent-Based Models – Best for simulating human behaviour, often applied in economic forecasting.
Watermark synthetic data – Clearly label it to prevent misuse and ensure transparency.
Test for bias – Regularly check if the synthetic dataset reinforces existing biases instead of correcting them.
Prioritise real data where possible – Synthetic data should complement, not replace real-world data for training AI models.
By following these steps, synthetic data can power smarter, fairer, and safer AI.
Comments